Abstract
Symmetric Archimedean copulas are widely applied for hydrologic analyses for the following reasons: (1) they can be easily constructed with the given generating function; (2) a large variety of copulas belong to this class (Nelsen, 2006); and (3) the Archimedean copulas have nice properties, such as simple and elegant mathematical treatment. This chapter focuses on the symmetric Archimedean copulas.
4.1 Definition of Symmetric Archimedean Copulas
Formally, a d-dimensional Archimedean symmetric copula Cd : [0, 1]d→[0, 1]Cd:01d→01 can be defined as follows (Nelsen, 2006; Salvadori et al, 2007; Savu and Trede, 2008). We first show it for a two-dimensional case and how it is constructed:

In Equation (4.1), ui, i = 1, 2,…,d, the marginal cumulative distribution function (CDF) of the ith random variable; and ϕ(⋅)ϕ⋅ is the generating function of the Archimedean copula, which has the following properties:
ϕ(⋅)ϕ⋅ is a continuous strictly decreasing function from [0, 1]→[0, ∞)01→0∞, we have ϕ(1) = 0ϕ1=0 and ϕ(0) = ∞ϕ0=∞, i.e., for ϕ(uk), k = 1, …, d; uk ∈ [0, 1], ϕ(uk) ∈ [0, ∞)ϕuk,k=1,…,d;uk∈01,ϕuk∈0∞.
ϕ[−1]ϕ−1 is the pseudo-inverse function of ϕϕ and nonincreasing on [0, ∞)0∞. ϕ[−1]ϕ−1 is strictly decreasing on [0, ϕ(0)]0ϕ0 with Domϕ[−1] ∈ [0, ∞)Domϕ−1∈0∞ and Ranϕ[−1] ∈ [0, 1]Ranϕ−1∈01 as follows:
ϕ−1=ϕ−1t;0≤t≤ϕ00;ϕ0≤t<∞(4.2)
ϕ[−1]ϕ−1 also has derivatives of all orders which alternate in sign, i.e., for all t to be in [0, ∞)0∞.
With k = 0, 1, …,k=0,1,…, it satisfies the following:
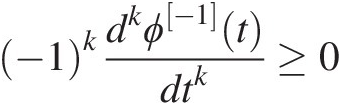
Following Equation (4.1), the two- and three-dimensional symmetric Archimedean copulas can be written as follows:
It should be noted that as the name of symmetric Archimedean copulas suggests, there is the same degree of dependence among all possible pairs for d ≥ 3d≥3. This fact usually hinders the application of symmetric Archimedean copulas for multivariate analysis in higher dimensions, since the dependence among the possible pairs in reality is usually not the same. We will illustrate it in subsequent chapters.
Example 4.1 Show that the function ϕ(t) = (− ln t)θ, θ ≥ 1ϕt=−lntθ,θ≥1 is the generating function of Archimedean copula, and express the corresponding two- and three-dimensional copulas with this generating function.
Solution: To show ϕ(t) = (− ln t)θ, θ ≥ 1ϕt=−lntθ,θ≥1 is the generating function of Archimedean copulas, we need to show that it is a continuous strict decreasing function.
1. Let f(t) = ln tft=lnt. It is obvious that f(t)ft is a strictly increasing function of t and thus −f(t) = − ln t−ft=−lnt is a strict decreasing function of t with − ln (0)→ ∞ , − ln (1) = 0.−ln0→∞,−ln1=0. Given θ ≥ 1θ≥1, we have (− ln (0))θ = ∞θ = ∞ ; (− ln (1))θ = 0∞ = 0−ln0θ=∞θ=∞;−ln1θ=0∞=0. Now we show ϕ(t) = (− ln t)θ, θ ≥ 1ϕt=−lntθ,θ≥1 satisfies the generating function ϕ(t) = (− ln t)θ, θ ≥ 1ϕt=−lntθ,θ≥1 is a continuous strictly decreasing from [0, 1]→[0, ∞)01→0∞.
2. The inverse of function ϕ(t)ϕt can be given as follows:
Let u = (− ln t)θ.u=−lntθ. Then we have ϕ−1t=exp(−u1θ)
. Now we need to show that ϕ−1(t)ϕ−1t is nonincreasing. And, it is obvious that the exponential function above is continuous and a nonincreasing function.
Applying Equation (4.1) for d = 2 or 3, we have the following:

and
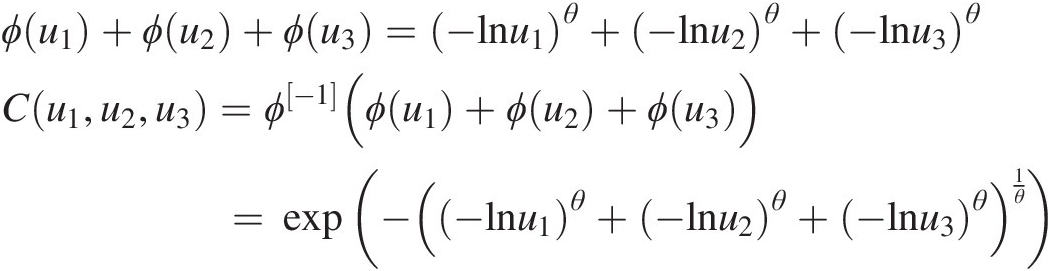
To illustrate the copula with the random variables in a real domain, let random variables {X1, X2, X3}X1X2X3 be positively dependent, and they may be modeled with the symmetric Archimedean copula in Equation (4.6b). In addition, X1, X2, X3X1,X2,X3 follow the marginal distributions, respectively, of
We then have u1=F1x1=1−exp−2×1,u2=F2x2=11+exp−x2−42,u3=Φx3−32. Now we have the following copula functions:
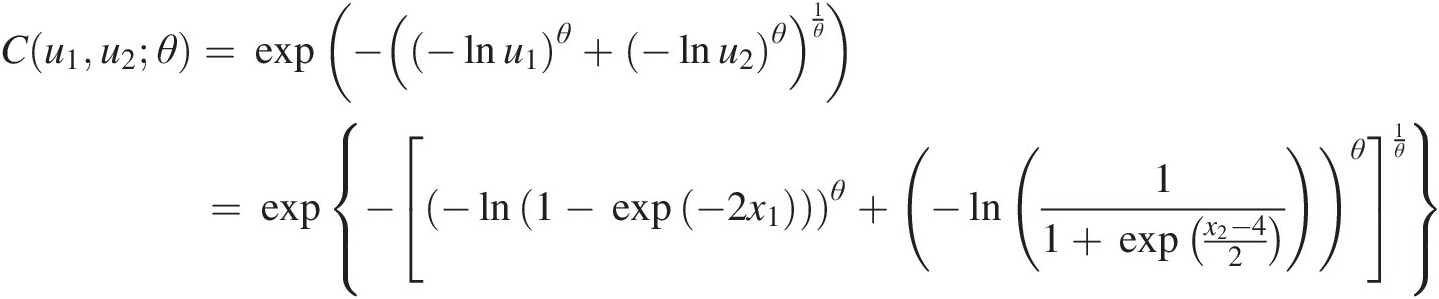

Equations (4.7a) and (4.7b) illustrate how to construct symmetric Archimedean copulas from the correlated random variables with following different marginal distributions. It may be worth noting here again that all cumulative marginal distribution ui~uniform (0, 1)ui~uniform01.
4.2 Properties of Symmetric Archimedean Copulas
Let C be an Archimedean copula with generating function ϕϕ. The Archimedean copula has the following properties (Nelsen, 2006; Salvadori et al., 2007; Savu and Trede, 2008):
C is permutation-symmetric in its d arguments. This indicates that the Archimedean copula is the distribution function of d exchangeable uniform random variates.
C is associative.
If α>0α>0 is any constant, then αϕαϕ is also a generator of C.
Example 4.2 Show for a given bivariate Archimedean copula function, one has C(u1, u2) = C(u2, u1)Cu1u2=Cu2u1.
Solution: Directly from Equation (4.1),
Example 4.3 Show that the copula is associative.
Suppose the symmetric Gumbel–Hougaard copula with parameter θ can be applied to study a given trivariate analysis. Show that the copula is associative, as follows:
Solution: The trivariate symmetric Gumbel–Hougaard copula can be expressed as follows:

in which ϕ(u) = (− ln u)θϕu=−lnuθ.
Now let’s prove the associative property of the symmetric copula using C(u1, C(u2, u3))Cu1Cu2u3 as an example. The inner copula function C(u2, u3)Cu2u3 is the bivariate Gumbel–Hougaard copula with the same parameter θ and can be written as follows:

Then C(u1, C(u2, u3))Cu1Cu2u3 is also the bivariate Gumbel–Hougaard copula and can be written as follows:

Finally, we have the following:

Similarly, we can prove that C(u1, u2, u3) = C(C(u1, u2), u3).Cu1u2u3=CCu1u2u3.
Equation (4.9) implies that given three random variables u1, u2, u3,u1,u2,u3, the dependence between the first two random variables taken together and the third one alone is the same as the dependence between the first random variable taken alone and the two last ones taken together. This implies a strong symmetry between different variables in that they are exchangeable (Malevergne and Sornette, 2006). But the associative property of the Archimedean copula is not satisfied by other copula families in general (Embrechts et al., 2001).
Example 4.4 Given the information u=12,v=14,w=16,andθ=12
, show that the associative property cannot be applied to the Farlie–Gumbel–Morgenstern copula.
Solution: The bivariate Farlie–Gumbel–Morgenstern copula can be expressed as follows:
With u=12,v=14,w=16,andθ=12, we have


and


Now we can reach the conclusion: C(u1, C(u2, u3))≠C(C(u1, u2), u3).Cu1Cu2u3≠CCu1u2u3.
Example 4.5 Using the bivariate Gumbel–Hougaard copula, show αϕαϕ is also a generator.
Solution: The generating function and the corresponding Gumbel–Hougaard copula can be written as follows:


in which θθ is the copula parameter.
For any given α, α>0α,α>0 and let ψ(t) = αϕ(t) = α(− ln t)θψt=αϕt=α−lntθ, we have the following:

Rearranging the preceding Gumbel–Hougaard copula function, we have the following:

Now, we show that αϕαϕ is also a generator of the Archimedean copula C, if α>0α>0.
Let U1, …, UdU1,…,Ud be d (dimensional) random variables with the joint distribution represented by the Archimedean copula C and generator ϕϕ. The distribution function of C(U1, …, Ud)CU1…Ud, i.e., Kendall distribution, KCKC, can be expressed as follows:
KCt=PCU1…Ud≤t=t+∑i=1d−1−1iϕiti!fi−1t(4.11)
where the auxiliary functions f0=1ϕ’t, and fi(t)fit for i ≥ 1i≥1 are defined recursively as fit=fi−1’tϕ’t
. For bivariate case, KCt=t−ϕtϕ’t
. An Archimedean copula is determined by the function KC(t)KCt defined on the unit interval [0,1]. This is a very useful result to determine which parametric copula family fits the data best (Savu and Trede, 2008).
From Section 3.4.2, we can derive the expression between Kendall’s τnτn and parameter of symmetric Archimedean copulas using K(t)Kt. Let bivariate random variables X and Y be modeled by the Archimedean copula, u = FX(x), v = FY(y).u=FXx,v=FYy. Then for the bivariate Archimedean copula C(u, v)Cuv, Equation (3.73) can be rewritten as follows:

Example 4.6 Consider the Gumbel–Hougaard copula with generator ϕ(t) = (− ln t)θ, θ ≥ 1ϕt=−lntθ,θ≥1. Derive Kendall’s τ from the Gumbel–Hougaard copula.
Solution: Taking the first derivative of ϕ(t)ϕt,ϕ’t=−θ−lntθ−1t,ϕtϕ’t=−lntθ−θ−lntθ−1t=tlntθ; Kendall’s ττ for the Gumbel–Hougaard copula is as follows:

Furthermore, in Equation (4.13) τ = 0τ=0 if θ = 1θ=1 (i.e., the bivariate random variable is independent), and the dependence increases with the increase of copula parameter θθ.
Example 4.7 Consider the Clayton copula with generator ϕt=t−θ−1θ
and parameter θ : θ ∈ [−1, ∞)\0θ:θ∈−1∞\0. Derive Kendall’s τ from the Clayton copula.
Solution: Taking the first derivative of ϕ(t)ϕt, we have the following:

Kendall’s ττ for the Clayton copula can then be computed as follows:

In Equation (4.14), τ = − 1τ=−1 when θ = − 1θ=−1 (i.e., perfectly negatively dependent). And τ→1τ→1 when θ→∞θ→∞ (i.e., perfectly positively dependent). Similar to Example 4.6, the dependence of the bivariate random variable increases with the increase of parameter θθ.
4.3 Archimedean Copula Families
4.3.1 Bivariate Archimedean Copula Families
There exists a large variety of symmetric Archimedean copula families that are used for constructing copulas to represent multivariate distributions. Table 4.1 lists the popularly applied one-parameter Archimedean copulas (Nelsen, 2006). Tables 4.2 and 4.3 list their first-order derivative of ∂∂u1Cu1u2 and the copula density c(u1, u2)cu1u2, respectively. One may refer to Nelsen (2006) for other one-parameter Archimedean copulas.
Table 4.1. Selected Archimedean copulas.
Name | Copula function Cθ(u1, u2)Cθu1u2 | Generating function ϕ(t)ϕt | Parameter θθ |
---|---|---|---|
Clayton | maxu1−θ+u2−θ−1−1θ0![]() | 1θt−θ−1![]() | [−1, ∞)\{0}−1∞\0 |
Ali–Mikhail–Haq | u1u21+1−u1θ1−u2θ1θ![]() | ln1−θ1−tt![]() | [−1, 1]−11 |
Gumbel–Hougaard | exp−−lnu1θ+−lnu2θ1θ![]() | (− ln t)θ−lntθ | [1, ∞)1∞ |
Frank | −1θln1+e−θu1−1e−θu2−1e−θ−1![]() | −lne−θt−1e−θ−1![]() | (−∞, ∞)\{0}−∞∞\0 |
Joe | 1−1−u1θ+1−u2θ−1−u1θ1−u2θ1θ![]() | − ln (1 − (1 − t)θ)−ln1−1−tθ | [1, ∞)1∞ |
Survival | u1u2e−θ ln u1 ln u2u1u2e−θlnu1lnu2 | ln(1 − θ ln t)ln1−θlnt | (0, 1]01 |
Table 4.2. First-order derivatives ∂∂u1Cθu1u2
for the selected Archimedean copulas.
Name | ∂∂u1Cθu1u2![]() |
---|---|
Clayton | u1−1−θ−1+u1−θ+u2−θ1+θθ,θ>0![]() |
Ali–Mikhail–Haq | u2+θu2−1+u2−1+θ−1+u1−1+u22![]() |
Gumbel–Hougaard | −lnu1−1+θ−lnu1θ+−lnu2θ−1+1θu1e−lnu1θ+−lnu2θ1θ![]() |
Frank | e−θu1e−θu2−1e−θu1+u2−e−θu1−e−θu2+e−θ![]() |
Joe | −1−u1−1+θ−1+1−u2θ1−u1θ+1−u2θ−1−u1θ1−u2θ−1+1θ![]() |
Survival | u2−θu2lnu2eθlnu1lnu2![]() |
Table 4.3. Copula density cθ(u1, u2)cθu1u2 for the selected Archimedean copulas.
∂2∂u1∂u2Cθu1u2![]() | |
Clayton | |
1+θu1−1−θu2−1−θ−1+u1−θ+u2−θ1+2θθ,θ>0![]() | |
Ali–Mikhail–Haq | |
−1+θ2−1+u2+u2−u1u2−θ−2+u1+u2−u1u2−1+θ−1+u1−1+u23![]() | |
Gumbel–Hougaard | |
−lnu2−1+θ−lnu1−1+θw2−2θθ−1−θw1−2θθu1u2ew1θ,w=−lnu1θ+−lnu2θ![]() | |
Frank | |
θeθ−1eθ1+u1+u2eθu1+u2−eθ1+u1−eθ1+u2+eθ2![]() | |
Joe | |
1−u11−u2−1+θθ−1+ww1θ−2![]() | |
Survival | |
1−θ−θlnu2+θlnu1−1+θlnu2eθlnu1lnu2![]() |
As discussed in Nelsen (2006), the Cook–Johnson (Clayton) family was derived by Clayton (1978), Oakes (1982, 1986), Cox and Oakes (1984), and Cook and Johnson (1981). This copula family can be used for modeling nonelliptically symmetric (nonnormal) multivariate data (Cook and Johnson, 1981). When θ = − 1θ=−1, the copula represents the joint distribution of the perfectly negatively dependent bivariate random variables, i.e., the Fréchet–Hoeffding lower bound: W : W = max (u1 + u2 − 1, 0)W:W=maxu1+u2−10. When θ = 0θ=0, the copula represents the joint distribution for independent bivariate random variables, i.e., product copula: Π : Π = u1u2Π:Π=u1u2. When θ→∞θ→∞, the copula represents the joint distribution for perfectly positively dependent bivariate random variables, i.e., the Fréchet–Hoeffding upper bound: M : M = min (u1, u2)M:M=minu1u2.
The Gumbel–Hougaard Archimedean copula was first introduced by Gumbel (1960). This copula family cannot be applied to model the negatively dependent bivariate random variables. Nelsen (2006) showed that the Gumbel–Hougaard copula belonged to the extreme value copula family. With this characteristic, the Gumbel–Hougaard Archimedean copula may be a suitable candidate for multivariate frequency analysis of extreme hydrological events, i.e., peak discharge and corresponding volume and duration.
The Ali–Mikhail–Haq Archimedean copula was developed by Ali et al. (1978). It was developed based on the concept of univariate logistic distribution that may be specified by considering a suitable form for the odds in favor of a failure against survival. The parameter of this copula is a measure of departure from independence or a measure of association between two random variables. In addition, the Ali–Mikhail–Haq copula can only capture the dependence within the range of τ ∈ [−0.182, 0.333]τ∈−0.1820.333, which limits the application of the Ali–Mikhail–Haq copula to bivariate frequency analysis.
The Frank Archimedean copula was developed by Frank (1979). The Frank copula satisfies all the conditions for the construction of bivariate distributions with fixed marginals except for independent variables (θ≠0)θ≠0), for the Frank copula). However, if the bivariate random variables are independent, the copula function is the product copula. Thus, the Frank copula is also considered absolutely continuous with full support on the unit square as the Cook–Johnson (Clayton) copula family.
The Joe Archimedean copula was first introduced by Joe (1993). When θ = 1θ=1, this copula represents the joint distribution for independent bivariate random variables. Similar to the Gumbel–Hougaard copula, the Joe copula cannot be applied to model negatively dependent bivariate random variables.
The survival copula is associated with Gumbel’s bivariate exponential distribution. This family is the survival copula that is actually the survival probability distribution of the Gumbel bivariate exponential distribution.
Example 4.8 Using the copulas given in Table 4.4, plot the density functions of bivariate Archimedean copulas. Can any conclusions be reached from these plots?
Table 4.4. Bivariate Archimedean copula parameters.
Copula | Parameter θθ | |
---|---|---|
Clayton | 0.5 | 2 |
Gumbel–Houggard | 2 | 5 |
Frank | –5 | 2 |
Joe | 2 | 5 |
Solution: With the corresponding copula density function listed in Table 4.3, Figure 4.1 plots the copula density functions for the copulas listed in Table 4.4. In the case of the Clayton copula, when θ ∈ [−1, 0)θ∈−10, its generating function is not strict. Thus, the Clayton copula is only sufficiently differentiable if θ>0θ>0. In addition, from Figure 4.1 and the discussion on tail dependence in Chapter 3, we can reach the following conclusions graphically: (1) the random variables are positively dependent and seem to have left (lower) tail dependence but no right (upper) tail dependence for the Clayton copula; (2) the random variables are positively dependent for the Gumbel–Hougaard and Joe copulas and exhibit the right (upper) tail dependence; and (3) the Frank copula does not seem to have either right (upper) or left (lower) tail dependence, and the random variables are negatively dependent when θ < 0θ<0.
Figure 4.1 Copula density plots.
4.3.2 Relation of Kendall’s τ and Parameter θ for Bivariate Archimedean Copulas
Equation (4.9) presents the relation between Kendall’s τ and the generating function of bivariate Archimedean copulas. In turn, the relation between Kendall’s τ and parameter θ for a given bivariate Archimedean copula can be determined. Table 4.5 lists this relation for the selected Archimedean copulas.
Table 4.5. Relations between ττ and θθ for selected symmetric Archimedean copulas.
Family | ϕ(t)ϕt | ϕ‘(t)ϕ′t | τ=1+4∫01ϕtϕ’tdt![]() | Range of ττ |
---|---|---|---|---|
Clayton | 1θt−θ−1![]() | −t−1 − θ−t−1−θ | θθ+2![]() | [−1, 1]\0−11\0 |
Ali–Mikhail–Haq | ln1−θ1−tt![]() | θ−1t−θt+θt2![]() | No analytical solution | −0.1813![]() |
Gumbel–Hougaard | (− ln t)θ−lntθ | −θt−lntθ−1![]() | 1−1θ![]() | [0, 1]01 |
Frank | −lne−θt−1e−θ−1![]() | θ1−eθt![]() | 1−4θD1−θ−1,![]() ![]() ![]() | [−1, 1]\0−11\0 |
Joe | − ln [1 − (1 − t)θ]−ln1−1−tθ | θ1−tθ−1−1+1−tθ![]() | No analytical solution | [0, 1] |
Survival | ln(1 − θ ln t)ln1−θlnt | θtlnt−1![]() | No analytical solution | [−0.3613, 0] |
4.4 Symmetric Multivariate Archimedean Copulas (d ≥ 3d≥3)
Nelsen (2006) stated that the bivariate Archimedean copula may not be extended to a multivariate case, unless additional conditions are satisfied to construct the symmetric multivariate Archimedean copulas to represent the joint distribution of multivariate random variables (i.e., d ≥ 3d≥3). Nelsen (2006) discussed the theorem and three additional useful results that are necessary to construct the appropriate multivariate Archimedean copula. These results are introduced in the following.
Theorem (Theorem 4.6.2, Nelsen, 2006): Let ϕϕ be a continuous strictly decreasing function from I to [0, ∞)0∞ with ϕ(0) = ∞ , ϕ(1) = 0ϕ0=∞,ϕ1=0, and ϕ−1ϕ−1 denote the inverse of ϕϕ. If Cd is the function from Id to I, given by Equation (4.1), then Cd is the copula function to represent the d-dimensional multivariate distribution if and only if ϕϕ is completely monotonic on [0, ∞)0∞, as follows:

Result 1: If function f is absolutely monotonic, i.e.,
dkf(x)/dxk ≥ 0, k = 0, 1, 2, …dkfx/dxk≥0,k=0,1,2,…(4.16)
and function g is completely monotonic, then the composite f ∘ gf∘g is completely monotonic.
Result 2: If functions f and g are completely monotonic, then so is their product fg.
Result 3: If f is completely monotonic and g is a positive function with a completely monotone derivative, then the composite f ∘ gf∘g is completely monotonic.
Table 4.6 lists the applicability to extend the selected bivariate Archimedean copula to higher dimension.
Table 4.6. Multivariate (d ≥ 3d≥3) symmetric Archimedean copulas.
Example 4.9 Show that the bivariate Clayton copula can be extended to higher dimension symmetric Clayton copulas for θ>0θ>0.
Solution: It is known that if the Clayton copula can be extended to higher dimensions, i.e., d ≥ 3d≥3, we need to satisfy the theorem (Theorem 4.6.2, Nelsen, 2006) discussed previously. The generating function for the Clayton copula can be written as follows:

According to Nelsen (2006), we know that for θ ≥ 0θ≥0, the generating function is strictly decreasing from I to (0, ∞)0∞. Applying Equation (4.15), we have the following:


…

Now, we reach the conclusion that the bivariate Clayton copula can be extended to multivariate symmetric Clayton copula, as follows:

Note that the multivariate symmetric Clayton copula (i.e., d ≥ 3d≥3) may only model the positive dependent/independent multivariate random variables. The reason is that if θ < 0θ<0, Equation (4.15) cannot be guaranteed to be fully satisfied.
Example 4.10 Show that the inverse of the generating function of the Ali–Mikhail–Haq copula is completely monotonic and thus the bivariate Ali–Mikhail–Haq copula can be extended to higher dimensions.
Solution: Following Nelsen (2006), it is known that the generating function of the Ali–Mikhail–Haq copula is strictly decreasing from I to (0, ∞)0∞. The generating function and its inverse function can be written as follows:

Rather than directly applying the theorem as in Example 4.9, here we use the inequality proposed by Widder (1941) for function ϕ−1ϕ−1 to be completely monotonic, as follows:
The first and second derivative of the inverse function can be written as follows:

Substituting the first and second derivatives of the inverse function into Equation (4.17), we have the following:
