Abstract
Much of the literature on copulas, discussed in the previous chapters, is limited to the bivariate cases. The Gaussian and student copulas have been commonly applied to model the dependence in higher dimensions (Genest and Favre, 2007; Genest et al., 2007a). In Chapter 4, we discussed the extension of symmetric bivariate Archimedean copulas as well as their major restrictions to model high-dimensional dependence (i.e., d ≥ 3)d≥3). Through the extension of the bivariate Archimedean copula, the multivariate Archimedean copula is symmetric and denoted as exchangeable Archimedean copula (EAC). EAC allows for the specification of only one generating function and only one set of parameters θ. In other words, random variates by pair share the same degree of dependence. Using the trivariate random variable {X1, X2, X3} as an example, {X1, X2}, {X2, X3}, and {X1, X3} should have the same degree of dependence. However, this assumption is rarely valid. This chapter discusses the following two approaches of constructing asymmetric multivariate copulas: nested Archimedean copula construction (NAC) and the vine copulas through pair-copula construction (PCC).
5.1 Construction of Higher-Dimensional Copulas
In general, there are dd−12 pairs of variables for a given d-dimensional multivariate problem. The NAC approach constitutes a significant improvement over EAC; however, it is still not rich enough to model all possible mutual dependencies among the d dimensional random variables (Berg and Aas, 2007). Based on the multivariate probability density function decomposition (Joe, 1997), the PCC approach allows for the free specification of dd−12
copulas that are hierarchical in nature. Further, it allows for selecting copulas from different families to model the dependence structure (Berg and Aas, 2007; Aas et al., 2009). Hence, the NAC approach is introduced first, followed by the PCC approach.
5.2 Nested Archimedean Copulas (NAC)
Representing one type of multivariate extension, NAC constitutes a significant improvement over EAC. We first review the fully nested Archimedean construction (FNAC) and the partially nested Archimedean construction (PNAC), and then turn to the general nested Archimedean copula.
5.2.1 Fully Nested Archimedean Copulas (FNAC)
For d-dimensional random variables modeled with FNAC, there are d – 1 bivariate copula functions, which result in dependence structure with partial exchangeability (Joe, 1997; Embrechts et al., 2003; Whelan, 2004; McNeil, 2007; Savu and Trede, 2010; among others). Figure 5.1 presents an example of a four-dimensional FNAC structure. The bivariate copula is the building block for FNAC. The FNAC structure is constructed, based on the degree of dependence between the pair variables, with the following procedures:
i. Choose the variables with the highest degree of dependence (rank-based) as the first two variables (1 and 2).
ii. Compute the empirical copula using variables 1 and 2.
iii. Evaluate the degree of dependence (rank-based) between empirical copula from step ii with the remaining variables.
iv. Choose variable 3, i.e., yielding the highest degree of dependence (rank-based) with the empirical copula built with variables 1 and 2.
v. Continue the process until the last variable is considered.
Figure 5.1 Four-dimensional FNAC structure.
From Figure 5.1, it is seen that three bivariate copulas are needed to represent the dependence for the four-dimensional random variables through FNAC as follows. First, random variables u1u1 and u2u2 are coupled through copula C3C3. Second, random variable u3u3 is coupled with C3(u1, u2)C3u1u2 through copula C2C2. Third, random variable u4u4 is coupled with C2(u3, C3(u1, u2))C2u3C3u1u2 through copula C1C1. Hence, a four-dimensional copula requires three bivariate copulas C1, C2C1,C2, and C3C3, with generators ϕ1ϕ1, ϕ2ϕ2, and ϕ3ϕ3 and may be written as follows:
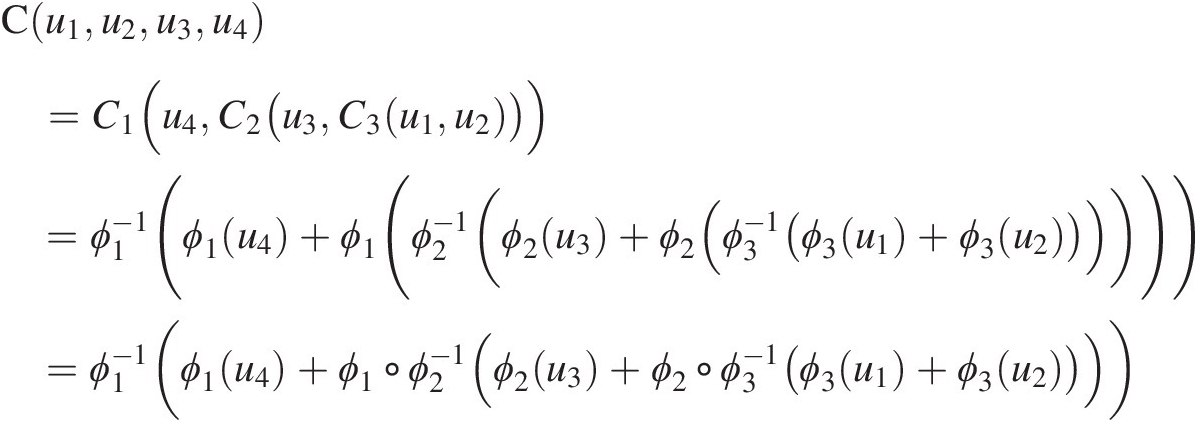
where ○ represents the composition of functions.
Similarly, the FNAC for d-dimensional random variables (e.g., Joe, 1997; Embrechts et al., 2003; Whelan, 2004; Nelsen, 2006) may be generated as follows:

It is worth noting that Equation (4.1) in Chapter 4, i.e., the exchangeable symmetric Archimedean copula, is a special case of Equation (5.2) if ϕ(θ1) = ϕ2(θ2) = … = ϕd − 1(θd − 1) = ϕ(θ), θ1 = θ2 = … = θd − 1ϕθ1=ϕ2θ2=…=ϕd−1θd−1=ϕθ,θ1=θ2=…=θd−1. For the d-dimensional FNAC, the bivariate margins themselves are also Archimedean copulas that allow for free specification of d – 1 copulas with the remaining identified implicitly through FNAC (Whelan, 2004; Berg and Aas, 2007). Using Equation (5.1) (Figure 5.1) as an example, this statement may be expressed as follows: (i) there are three Archimedean copulas of free specification, i.e., C3C3 with parameter θ3θ3 for variables u1, u2u1,u2; C2C2 with parameter θ2θ2 for variables {u3, C3(u1, u2; θ3}{u3,C3(u1,u2;θ3}; and C1C1 with parameter θ1θ1 for variables {u4, C2(u3, C3(u1, u2; θ3); θ2}{u4,C2(u3,C3u1u2θ3;θ2}; (ii) pairs (u1, u3), (u2, u3)u1u3,u2u3 have copula C2C2 with parameter θ2θ2; and (iii) pairs (u1, u4), (u2, u4), (u3, u4)u1u4,u2u4,u3u4 have copula C3C3 with parameter θ1θ1. The decreasing degree of dependence for the increasing levels of nesting (i.e., θ1 ≤ θ2 ≤ … ≤ θd − 1θ1≤θ2≤…≤θd−1 with θ1θ1 and θd − 1θd−1 representing the parameters for the highest and lowest levels, respectively) is another technical condition for proper construction of the d-dimensional fully nested asymmetric Archimedean copula.
It should also be pointed out that the following conditions need to be satisfied for the nested generating functions:
ϕ1−1,ϕ2−1,…,ϕd−1−1
must satisfy the necessary conditions for being completely monotonic.
According to Embrechts et al. (2003), the coupling of functions wk=ϕk∘ϕk+1−1
belongs to a class of functions ℒ∞∗
defined as follows:
ℒ∞∗=ω:0∞→0∞ω0=0ω∞=∞−1k−1dkωtdt≥0k=12…∞(5.3)
Based on Equation (5.2), the simplest three-dimensional FNAC (shown in Figure 5.2) can be written as follows:

Figure 5.2 Three-dimensional FNAC structure.
In accordance with Equation (5.4), we outline here the derivation of five three-dimensional asymmetric Archimedean copulas that are commonly applied.
M3 (Joe, 1997):

Let t = C2(u1, u2)t=C2u1u2. Then we have C1u3t=−1θ1ln1−1−e−θ1u3(1−e−θ1t1−e−θ1

θ2 ≥ θ1 ∈ [0, ∞), τ12, τ13, τ23 ∈ [0, 1]θ2≥θ1∈0∞,τ12,τ13,τ23∈01 for positive dependent trivariate variables.
The M3 copula may be also called the asymmetric trivariate Frank copula.
We now use the following specific examples to illustrate these marginal distributions.
Example 5.1 Derive the M3 copula for θ1 = 2.0 and θ2 = 3.0θ1=2.0andθ2=3.0 by setting u3 = 0.6u3=0.6. Assuming u1~F1(x1) : X1~gamma(2, 4); u2~F2(x2) : X2~normal(1, 32); u3~F3(x3) : X3~EV1(10, 7)u1~F1x1:X1~gamma24;u2~F2x2:X2~normal132;u3~F3x3:X3~EV1107, and {X1, X2}X1X2 has a higher pairwise dependence.
Solution: With {X1, X2}X1X2 having higher pairwise dependence, we first couple X1and X2X1andX2 and build the copula function from the marginals as follows:



Since we already set u3 = 0.6u3=0.6, then we have x3≈9.388x3≈9.388 from the EV1 population.
Finally, we can write the fully nested copula using the M3 copula as follows:


Figure 5.3(a) plots the corresponding joint CDF for the derived M3 copula with u3 = 0.6u3=0.6. M4 (Joe, 1997):

Let t = C2(u1, u2)t=C2u1u2. Then we have C1u3t=u3−θ1+t−θ1−1−1θ1

θ2 ≥ θ1 ∈ [0, ∞), τ12, τ13, τ23 ∈ [0, 1]θ2≥θ1∈0∞,τ12,τ13,τ23∈01 for positive dependent trivariate variables. The M4 copula may also be called the trivariate asymmetric Clayton copula.
Figure 5.3 Joint CDF for derived FNACs: (a) M3 copula, (b) M4 copula, (c) M5 copula, (d) M6 copula, and (e) M12 copula.
Example 5.2 Derive the M4 copula using information given in Example 5.1.
Solution: In Example 5.1, we have θ1 = 2.0, θ2 = 3.0θ1=2.0,θ2=3.0 by setting u3 = 0.6u3=0.6. Thus, we have the following:


Figure 5.3(b) plots the corresponding joint CDF for the derived M4 copula with u3 = 0.6u3=0.6.
M5 (Joe, 1997):

Let t=C2u1u2,1−t=1−u1θ2+1−u2θ2−1−u1θ21−u2θ21θ2. Then we have the following:

θ2 ≥ θ1 ∈ [1, ∞), τ12, τ13, τ23 ∈ [0, 1]θ2≥θ1∈1∞,τ12,τ13,τ23∈01. The M5 copula may also be called the trivariate asymmetric Joe copula.
Example 5.3 Derive M5 copula using the information given in Example 5.1.
Solution: In Example 5.1, we have θ1 = 2.0, θ2 = 3.0θ1=2.0,θ2=3.0 by setting u3 = 0.6u3=0.6. Thus we have the following:



Figure 5.3(c) plots the corresponding joint CDF for the derived M5 copula with u3 = 0.6u3=0.6.
M6 (Joe, 1997; Embrechts, 2003):
Let C2u1u2=e−−lnu1θ2+−lnu2θ21θ2,and


θ2 ≥ θ1 ∈ [1, ∞), τ12, τ13, τ23 ∈ [0, 1]θ2≥θ1∈1∞,τ12,τ13,τ23∈01 for positive dependent trivariate variables. The M6 copula may also be called the trivariate asymmetric Gumbel–Hougaard copula.
Example 5.4 Derive the M6 copula using the information given in Example 5.1.
Solution: In Example 5.1, we have θ1 = 2.0, θ2 = 3.0θ1=2.0,θ2=3.0 by setting u3 = 0.6u3=0.6. Thus we have the following:


Figure 5.3(d) plots the corresponding joint CDF for the derived M6 copula with u3 = 0.6u3=0.6.
M12 (Embrechts, 2003):

Let t=C2u1u2,1t−1=1u1−1θ2+1u2−1θ21θ2. Then we have


Example 5.5 Derive the M12 copula using the information given in Example 5.1.
Solution:


Figure 5.3(e) plots the joint CDF for the derived M12 copula with u3 = 0.6u3=0.6.
Solution: From Figure 5.1, we have the following:

C3C3 and u3u3 are coupled as copula C2(C3, u3)C2C3u3 with parameter θ2θ2, which can be written as follows:

Finally, C2C2 and u4u4 are defined as copula C1(C2, u4)C1C2u4 with parameter θ1θ1, which results in C1(C2, u4; θ1) = C(u1, u2, u3, u4; θ1, θ2, θ3)C1C2u4θ1=Cu1u2u3u4θ1θ2θ3 as follows:

In the same way as for the previous examples, for the four-dimensional random variables {Xi, i = 1, …, 4}Xii=1…4, the random variable XiXi may follow different marginal distributions as follows:



5.2.2 Partially Nested Archimedean Copulas (PNAC)
Originally, Joe (1997) proposed the structure of PNAC as an alternative approach for FNAC. PNAC may be considered a composite of EAC and FNAC (Berg and Aas, 2007).Similar to FNAC, PNAC also has d – 1 bivariate copulas that are partially exchangeable. As a simple example, Figure 5.4 illustrates the PNAC structure for four-dimensional random variables: (1) couple the two pairs (u1, u2)u1u2 and (u3, u4)u3u4 with copula C3C3 with parameter θ3θ3 and C2C2 with parameter θ2,θ2, respectively, at the first level; and (2) the third copula C1C1 with parameter θ1θ1 will be applied to couple C2C2 and C3C3 at the second level (Berg and Aas, 2007). Figure 5.4 also shows (1) exchangeability between u1u1 and u2u2, as well as between u3u3 and u4u4; and (2) four pairs (u1, u3), (u1, u4), (u2, u3)u1u3,u1u4,u2u3, and (u2, u4)u2u4 all have copula C1C1. Furthermore, the same constraints on parameters for FNAC are required to be satisfied for PNAC (Berg and Aas, 2007), i.e., (i) PNAC may be used to model the positively dependent variables, and (ii) the dependence decreases with the increase of nesting levels (i.e., the parameters of a higher level are smaller than those of a lower level).
Figure 5.4 Partially nested Archimedean construction.
Example 5.7 Using the bivariate Frank copula as the building block to derive a four-dimensional PNAC function for the structure given in Figure 5.4.
Solution: As shown in Figure 5.4, (u1, u2)u1u2 and (u3, u4)u3u4 can be represented through the Frank copula as follows:


Then C1C1 can be represented through C3, C2C3,C2 as follows:

with the parameters: 0 ≤ θ1 ≤ θ2, θ30≤θ1≤θ2,θ3.
In the same manner for FNAC, random variables {X1 : i = 1, 2, 3, 4}X1:i=1234 may follow different marginal distributions as ui = Fi(xi)ui=Fixi.
5.2.3 General Case
Originating in Joe (1997), the general nested Archimedean copula (GNAC) construction was further developed by Whelan (2004) and Savu and Trede (2006). Savu and Trede (2006) first introduced the notation for arbitrary nesting and the procedure for calculating the d-dimensional probability density function in general. To build a hierarchy of Archimedean copulas, they also applied the notation for the hierarchical Archimedean copula for GNAC. The main idea of the generally nested Archimedean construction is presented in this section (Berg and Aas, 2007).
For the GNAC with L levels, there are nlnl distinct objects (an object is either a copula or a variable) at each level l. At level l = 1l=1, variables u1, …, udu1,…,ud are grouped into n1n1 exchangeable multivariate Archimedean copulas. These copulas are, in turn, coupled with n2n2 copula at level l = 2l=2, and so on. Berg and Aas (2007) presented an example of a nine-dimensional copula to explain this structure (Figure 5.5).
Figure 5.5 Hierarchically nested Archimedean copula construction.
Following Figure 5.5, the nine-dimensional copula can be written as
At the first level, there are two two-dimensional EACs, i.e., C41(u1, u2)C41u1u2 with parameter θ41θ41 and C42(u8, u9)C42u8u9 with parameter θ42θ42. There are one three-dimensional and one two-dimensional EACs at the second level, i.e., C31(C41, u3, u4)C31C41u3u4 with parameter θ31θ31 and C32(u7, C42)C32u7C42 with parameter θ32θ32. At the third level, there is only one copula, C21(C31, u5, u6)C21C31u5u6 with parameter θ21θ21. At the top (fourth) level, the copula C11C11, with parameter θ11θ11, is applied to model the dependence between C21C21 and C32C32.
To ensure that GNAC is a valid Archimedean copula, there are a number of conditions that need to be satisfied (Savu and Trede, 2006; Berg and Aas, 2007):
a. The number of copulas must decrease with the increasing level of nesting. The top level may contain only one copula, and the inverse of the generating functions (ϕ−1ϕ−1) must be completely monotonic.
b. The dependence of GNAC must decrease with the increasing level of nesting. For example, in Figure 5.5, parameters must be stratified following the condition θ41 ≥ θ32 ≥ θ21 ≥ θ11θ41≥θ32≥θ21≥θ11 and θ42 ≥ θ32 ≥ θ11θ42≥θ32≥θ11. However, when mixing copula generators that belong to different Archimedean copula families, this requirement might not be sufficient. Two Archimedean copulas from different families (i.e., Fam1 and Fam2) can only be nested if the derivative of the product ϕ1∘ϕ2−1
is completely monotonic. Joe (1997) presented details about copula families that can be mixed and explored structures where all the generators are from the same family are explored, and the other structures are still not fully explored.
5.2.4 Parameter Estimation for Nested Copulas
For NAC with an explicit density expression, the maximum likelihood estimation method is commonly applied to estimate the copula parameters; however, the NAC density function may not be straightforwardly derived. Savu and Trede (2006) proposed a recursive approach to derive the density function for general NAC. With this approach, the number of computational steps for evaluating the density increases rapidly with the copula complexity, and parameter estimation becomes very time consuming in higher dimensions (Savu and Trede, 2006; Berg and Aas, 2007).
The density function of NAC can be derived using the chain rule as discussed by Savu and Trede (2006). We will use the following examples to illustrate the general procedure on how to apply the chain rule. Furthermore, we derive the density functions for the M3, M4, M5, M6, and M12 copulas (Joe, 1997) in the appendix as specific examples.
Example 5.8 Derive the density function for three-dimensional FNAC (Equation (5.4) corresponding to Figure 5.2).
Solution: Equation (5.4) may be rewritten as follows:
C(u1, u2, u3) = C1(C2(u1, u2), u3)Cu1u2u3=C1C2u1u2u3 and its density, i.e., c(u1, u2, u3)cu1u2u3, may be derived as follows:

Finally, we have the following:

Example 5.9 Derive the density function for four-dimensional FNAC (i.e., Equation (5.1) corresponding to Figure 5.1).
Solution: Following Equation (5.1) and Figure 5.1, we have the following:
C(u1, u2, u3, u4) = C1(u4, C2) = C1(u4, C2(u3, C3(u1, u2)))Cu1u2u3u4=C1u4C2=C1u4C2u3C3u1u2 and its density c(u1, u2, u3, u4)cu1u2u3u4 may be derived as follows:

Finally, we have the following:

Example 5.10 Derive the density function for the copula function represented by Figure 5.4.
Solution: According to Figure 5.4, we have the following: C(u1, u2, u3, u4) = C1(C3(u1, u2), C2(u3, u4)).Cu1u2u3u4=C1C3u1u2C2u3u4. Then its density function c(u1, u2, u3, u4)cu1u2u3u4 may be expressed as follows:



Finally, we have the following:

With the copula density function derived, we can then apply MLE to estimate parameters simultaneously with the constraints of parameters at a lower level being larger than those at a higher level. However, the copula parameters may also be estimated sequentially with the use of MLE as follows:
i. Estimate the copula parameter at the lowest level.
ii. Estimate the copula parameter for the second-lowest level by fixing the parameters estimated for the lowest level.
iii. Repeat the preceding steps until we reach the top level of the NAC structure.
5.2.5 Simulation for Nested Copulas
In the previous chapters, we have shown that EAC may be simulated with several methods, such as Laplace transform (LT) and CPI Rosenblatt’s transform, and through its unique generating function ϕϕ with a simple algorithm. Frees and Valdez (1998) showed how to use the LT method to simulate NACs for the generators taken from either the Gumbel– Hougaard or the Clayton copula family. However, Berg and Aas (2007) have pointed out that the LT method is limited to the copulas such that we can find a distribution that equals the LT of the inverse generating function and from which we can easily sample. In most cases, the LT method needs to obtain the d – 1 first derivatives of the copula function, which usually yield extremely complex expressions under higher-order derivatives. The limitation of LT method may cause the simulation to become inefficient for high dimensions (Berg and Aas, 2007).
Compared to the LT method, the CPI Rosenblatt transform method is more universal and will be introduced to simulate from NAC. Let X = {X1, X2, …, Xd}X=X1X2…Xd be a d-dimensional random vector with marginal distributions F(xi)Fxi and conditional distributions F(xi| x1, …, xi − 1), i = 1, …, dFxix1…xi−1,i=1,…,d. The CPI Rosenblatt’s transform of X is defined as T(X) = {T(X1), …, T(Xd)}TX=TX1…TXd:
With the use of CPI method, random variables are simulated with the following procedure:
i. Generate W = {w1, w2, …, wd}W=w1w2…wd independent random variables following the uniform distribution [0, 1].
ii. Set x1 = w1x1=w1.
iii. Set w2 = T(X2) = F2 ∣ 1(x2| x1)w2=TX2=F2∣1x2x1 to obtain x2=F2∣1−1w2x1.
iv. Set w3 = T(X3) = F3 ∣ 1, 2(w3| x1, x2)w3=TX3=F3∣1,2w3x1x2 to obtain x3=F3∣1,2−1w3x1x2
.
…
Set wd = T(Xd) = Fd ∣ 1, 2, …d − 1(wd| x1, x2, …, xd)wd=TXd=Fd∣1,2,…d−1wdx1x2…xd.
Example 5.11 Assuming the pseudo-observations given in Table 5.1 may be modeled with the M6 copula, (1) estimate the copula parameters both simultaneously and sequentially using MLE; and (2) simulate the random variables with a sample size of 50.
Table 5.1. Trivariate pseudo-observations.
u1u1 | u2u2 | u3u3 | |
---|---|---|---|
1 | 0.241 | 0.138 | 0.103 |
2 | 0.241 | 0.172 | 0.172 |
3 | 0.241 | 0.241 | 0.276 |
4 | 0.241 | 0.586 | 0.655 |
5 | 0.793 | 0.828 | 0.897 |
6 | 0.483 | 0.345 | 0.379 |
7 | 0.931 | 0.914 | 0.621 |
8 | 0.724 | 0.759 | 0.724 |
9 | 0.414 | 0.621 | 0.586 |
10 | 0.759 | 0.414 | 0.310 |
11 | 0.862 | 0.793 | 0.793 |
12 | 0.655 | 0.517 | 0.448 |
13 | 0.414 | 0.379 | 0.552 |
14 | 0.569 | 0.448 | 0.414 |
15 | 0.569 | 0.690 | 0.690 |
16 | 0.414 | 0.310 | 0.241 |
17 | 0.241 | 0.552 | 0.862 |
18 | 0.069 | 0.035 | 0.035 |
19 | 0.241 | 0.276 | 0.345 |
20 | 0.069 | 0.069 | 0.069 |
21 | 0.897 | 0.914 | 0.931 |
22 | 0.655 | 0.655 | 0.483 |
23 | 0.069 | 0.103 | 0.138 |
24 | 0.241 | 0.207 | 0.207 |
25 | 0.655 | 0.724 | 0.759 |
26 | 0.517 | 0.483 | 0.517 |
27 | 0.828 | 0.862 | 0.828 |
28 | 0.966 | 0.966 | 0.966 |
Solution: Estimate the copula parameters.
To estimate the parameters for the fitted M6 copula, we use Figure 5.2 as the FNAC scheme.
Estimate the copula parameters simultaneously.
To estimate the copula parameters simultaneously, the copula density function (i.e., Equation (M6–3) in the appendix) is applied to write the log-likelihood function as follows:
logL=∑1u1u2u3−lnu1θ2−1−lnu2θ2−1−lnu3θ3−1e−w1θ1(G2θ1θ2−2w3θ1−3+2θ1−2w2θ1−2+θ2−θ1Gθ1θ2−2w2θ1−2+θ1−12θ1−1G2θ1θ2−2w1θ1−3+θ1−1G2θ1θ2−2w2θ1−3+θ1−1θ2−θ1Gθ1θ2−2w1θ1−2)where G =−lnu1θ2+−lnu2θ2;w=−lnu3θ1+[−lnu1θ2+−lnu2)θ1θ1θ2.
The parameter constraint is given as 1 ≤ θ1 ≤ θ21≤θ1≤θ2, where θ2θ2 corresponds to the parameters for the first level.
Maximizing the log-likelihood function numerically (e.g., using genetic algorithm ga function in MATLAB), the parameters are estimated as follows:
θ2 = 4.4158; θ1 = 3.3532θ2=4.4158;θ1=3.3532.
It is worth noting that to properly estimate the parameters simultaneously, the linear constraint needs to be applied with vector A = [–1,1] B = 0, which represents –θ2 + θ1 ≤ 0–θ2+θ1≤0.
Estimate the copula parameters sequentially.
To estimate the copula parameters sequentially, the density function for the bivariate Gumbel–Hougaard copula is applied (Chapter 4).
Step 1: Maximizing the log-likelihood function for (u1, u2)u1u2, we have θ2 = 4.4682θ2=4.4682.
Step 2: Compute C(u1, u2; θ2 = 4.4682C(u1,u2;θ2=4.4682) and estimate the parameter for
(u3, C(u1, u2; θ2 = 4.4682))u3Cu1u2θ2=4.4682. Again using MLE, we have θ1 = 3.2088θ1=3.2088. It is worth noting that to estimate the parameter (i.e., the Gumbel–Hougaard copula) for the top level, the lower and upper bounds are [1, θ2]1θ2.
Finally, for both simultaneous and sequential estimation, the parameters estimated are coded as follows:
param = [param(1), param(2)] = [θ2, θ1]param=param1param2=θ2θ1; param(1) and param(2) represents bottom and top levels, respectively.
Simulation from the fitted M6 copula.
As discussed previously, the random variates are simulated using the CPI Rosenblatt transform, as shown in Figure 5.6(a).
In addition, we have discussed previously that [u1, u3]u1u3 and [u2, u3]u2u3 may be modeled with the Gumbel–Hougaard copula with parameter θ1θ1. Figure 5.6(b) compares the simulation as well as the box plot of simulated and sample Kendall’s tau (100 simulations with a sample size of 28).
Figure 5.6 (a) Comparison of pseudo-observations with those simulated from M6 copula; (b) simulation comparison from the Gumbel–Hougaard copula with parameter θ1θ1 for (u1, u3), (u2, u3)u1u3,u2u3 directly; (c) comparison of sample Kendall’s tau with simulated Kendall’s tau from Gumbel–Hougaard copula with parameter θ = 2.8816θ=2.8816.
Example 5.12 Assuming the Gumbel–Hougaard copula may be applied as a biviarate building block, and using the scheme shown in Figure 5.4 and the pseudo-observations listed in Table 5.2, (1) estimate the copula parameters; and (2) simulate random variates with fitted copula for a sample size of 100.
Table 5.2. Pseudo-observations for Example 5.12.
u1u1 | u2u2 | u3u3 | u4u4 | |
---|---|---|---|---|
1 | 0.194 | 0.338 | 0.421 | 0.545 |
2 | 0.819 | 0.901 | 0.743 | 0.705 |
3 | 0.614 | 0.639 | 0.615 | 0.662 |
4 | 0.235 | 0.208 | 0.298 | 0.292 |
5 | 0.792 | 0.755 | 0.865 | 0.894 |
6 | 0.433 | 0.517 | 0.559 | 0.480 |
7 | 0.130 | 0.197 | 0.095 | 0.087 |
8 | 0.570 | 0.583 | 0.802 | 0.680 |
9 | 0.128 | 0.274 | 0.256 | 0.137 |
10 | 0.218 | 0.116 | 0.262 | 0.481 |
11 | 0.468 | 0.367 | 0.367 | 0.439 |
12 | 0.490 | 0.434 | 0.391 | 0.515 |
13 | 0.194 | 0.083 | 0.019 | 0.042 |
14 | 0.120 | 0.227 | 0.178 | 0.289 |
15 | 0.676 | 0.601 | 0.759 | 0.673 |
16 | 0.990 | 0.990 | 0.991 | 0.993 |
17 | 0.657 | 0.777 | 0.942 | 0.950 |
18 | 0.226 | 0.174 | 0.284 | 0.134 |
19 | 0.828 | 0.857 | 0.836 | 0.916 |
20 | 0.373 | 0.367 | 0.151 | 0.249 |
21 | 0.698 | 0.656 | 0.727 | 0.584 |
22 | 0.645 | 0.738 | 0.641 | 0.787 |
23 | 0.025 | 0.051 | 0.034 | 0.199 |
24 | 0.298 | 0.300 | 0.470 | 0.394 |
25 | 0.906 | 0.936 | 0.955 | 0.950 |
26 | 0.658 | 0.476 | 0.556 | 0.647 |
27 | 0.302 | 0.158 | 0.224 | 0.105 |
28 | 0.581 | 0.393 | 0.733 | 0.779 |
29 | 0.371 | 0.433 | 0.179 | 0.145 |
30 | 0.169 | 0.537 | 0.213 | 0.344 |
31 | 0.041 | 0.083 | 0.009 | 0.059 |
32 | 0.982 | 0.978 | 0.928 | 0.935 |
33 | 0.585 | 0.162 | 0.326 | 0.312 |
34 | 0.618 | 0.753 | 0.661 | 0.633 |
35 | 0.280 | 0.622 | 0.400 | 0.574 |
36 | 0.902 | 0.969 | 0.879 | 0.904 |
37 | 0.440 | 0.648 | 0.587 | 0.811 |
38 | 0.243 | 0.147 | 0.281 | 0.524 |
39 | 0.044 | 0.081 | 0.177 | 0.052 |
40 | 0.122 | 0.149 | 0.229 | 0.180 |
41 | 0.497 | 0.645 | 0.528 | 0.545 |
42 | 0.701 | 0.644 | 0.745 | 0.599 |
43 | 0.323 | 0.538 | 0.806 | 0.796 |
44 | 0.013 | 0.044 | 0.063 | 0.041 |
45 | 0.651 | 0.721 | 0.774 | 0.646 |
46 | 0.190 | 0.298 | 0.773 | 0.841 |
47 | 0.520 | 0.772 | 0.636 | 0.542 |
48 | 0.926 | 0.943 | 0.900 | 0.812 |
49 | 0.468 | 0.447 | 0.518 | 0.633 |
50 | 0.868 | 0.894 | 0.893 | 0.905 |
51 | 0.422 | 0.710 | 0.727 | 0.560 |
52 | 0.888 | 0.835 | 0.868 | 0.823 |
53 | 0.372 | 0.590 | 0.734 | 0.792 |
54 | 0.132 | 0.116 | 0.095 | 0.041 |
55 | 0.429 | 0.288 | 0.219 | 0.125 |
56 | 0.390 | 0.366 | 0.375 | 0.172 |
57 | 0.983 | 0.986 | 0.991 | 0.990 |
58 | 0.980 | 0.988 | 0.976 | 0.974 |
59 | 0.308 | 0.318 | 0.147 | 0.193 |
60 | 0.932 | 0.913 | 0.943 | 0.933 |
Solution:
1. Estimate the copula parameters.
According to Figure 5.4, let us use θ12, θ34θ12,θ34 to represent the copula parameters of [u1, u2], [u3, u4]u1u2,u3u4 at the bottom level and θθ to represent the copula parameter at the top level.
Estimate the parameters simultaneously.
Given the Gumbel–Hougaard copula as a bivariate building block, the copula density function for the four-dimensional PNAC Gumbel–Hougaard copula may be derived based on the chain rule following the procedure given in Example 5.10. With the parameter constraints 1 ≤ θ ≤ θ12, θ341≤θ≤θ12,θ34, i.e.,
θ−θ12≤0θ−θ34≤0
, the inequality vector is then given as A=−1,0,10,−1,1,B=00
, with the parameter set as param = [θ12, θ34, θ]param=θ12θ34θ.
The parameters can be estimated numerically by maximizing the log-likelihood function with the preceding linear constraint as follows:
θ12 = 3.6949, θ34 = 4.5035, θ = 2.8816θ12=3.6949,θ34=4.5035,θ=2.8816.
Estimate the parameters sequentially.
With the same estimation procedures shown in Example 5.11:
The parameter for (u1, u2)u1u2 is estimated as θ12 = 3.8545θ12=3.8545.
The parameter for (u3, u4)u3u4 is estimated as θ34 = 4.3949θ34=4.3949.
The parameter for {C3(u1, u2; θ12), C2(u3, u4; θ34)}C3u1u2θ12C2u3u4θ34 is estimated by fixing θ12, θ34θ12,θ34 as θ = 3.3297θ=3.3297.
2. Simulate random variates.
Using the CPI Rosenblatt transform, Figure 5.7(a) compares the pseudo-observations in Table 5.2 with those simulated from the fitted PNAC Gumbel–Hougaard copula function.
Figure 5.7 (a) Comparison of pseudo-observations with those simulated with the parameters estimated simultaneously (θ12 = 3.6949, θ34 = 4.5035, θ = 2.8816θ12=3.6949,θ34=4.5035,θ=2.8816); (b) comparison of observed variables with simulated variables with θ = 2.8816θ=2.8816; (c) comparison of sample Kendall’s tau with the simulated Kendall’s taus.
As discussed previously for the PNAC structure, we know (u1, u3), (u1, u4), (u2, u3), (u2, u4)u1u3,u1u4,u2u3,u2u4 should have the same joint distribution that may be modeled using the Gumbel–Hougaard copula with parameter at the top level, i.e., θ = 2.8816θ=2.8816 with the comparison of simulated random variable and Kendall’s tau as shown in Figure 5.7(b) and 5.7(c). Figure 5.7(b) and 5.7(c) indicates that the preceding four pairs may be modeled using the same Gumbel–Hougaard copula.
5.3 Pair-Copula Construction (PCC)
PCCs are also hierarchical in nature. Compared to EAC and NAC, a large improvement is made in PCCs that allows for the free specification of dd−12 copulas. The modeling scheme of PCCs is based on the decomposition of a multivariate density function. The d-dimensional probability density function may be decomposed to dd−12
bivariate density functions, where the first d − 1d−1 density functions are unconditional and the rest are conditional (Berg and Aas, 2007). First proposed by Joe (1997), there are two main types of PCCs, canonical (C)-vines and D-vines, in the literature (e.g., Bedford and Cooke, 2001, 2002; Kurowicka and Cooke, 2004, 2006; Aas et al., 2009).
5.3.1 Principle of Pair-Copula Decomposition of General Multivariate Distribution
Following Aas et al. (2009), we introduce the pair-copula decomposition of general multivariate distributions.
Let X = (X1, X2, …, Xd)X=X1X2…Xd be a vector of random variables with a joint density function f(x1, …, xd)fx1…xd. According to the conditional probability theory, the joint density function can be defined as follows:
In Chapters 3 and 4, the multivariate distribution F with marginals F1(x1), …, Fd(xd)F1x1,…,Fdxd is defined using Sklar’s theorem as follows:

where ui = Fi(xi)ui=Fixi; Fi−1ui is the inverse distribution of marginal Fi(xi)Fixi.
Then, for an absolutely continuous F with strictly increasing, continuous marginal probability densities f1(x1), …, fd(xd)f1x1,…,fdxd, applying ∂d∂x1…∂xd to Equation (5.13), we have


where c1, 2, …, d(⋅)c1,2,…,d⋅ stands for the d-dimensional copula density function.
In the bivariate case, Equation (5.14b) can be simplified to
where c12(⋅)c12⋅ is the appropriate pair-copula density.
Using the conditional probability in Equation (5.12), the conditional probability density function can be easily written as

Likewise, we have
Similarly, in the trivariate case, we can obtain the conditional probability density function:

According to the definition of conditional copula, we have

Thus,

Alternatively, f(x1| x2, x3)fx1x2x3 may be also written as follows:
Equations (5.20) and (5.21) can be further decomposed as follows:
From the expression of the appropriate pair-copula, a conditional marginal density function can be expressed in a general form as follows:
where v is a d-dimensional vector; vjvj is one arbitrarily chosen component of v; and v−jv−j denotes the v vector except vjvj, i.e., v−j = v\vjv−j=v\vj.
Under appropriate conditions, a multivariate probability density function may be expressed through the product of pair-copulas, acting on several different conditional probability distributions (Aas et al., 2009).
Joe (1997) showed a conditional marginal distribution for the appropriate pair-copula for every j as

where Cx, vj ∣ v−jCx,vj∣v−j is a bivariate copula function with the conditional marginals. For the special case where v is univariate, Equation (5.24) can be rewritten as follows:

In Equation (5.25), when xx and vv are copula random variables (i.e., the margins following the uniform [0,1] as f(x) = f(v) = 1, FX(x) = x, FV(v) = vfx=fv=1,FXx=x,FVv=v), Equation (5.25) can be rewritten as follows:

where the second variable of h(⋅)h⋅ function represents the conditional variable, and ΘΘ denotes the set of copula parameters to model the joint distribution function of xx and vv. Letting u = xu=x, Equation (5.26) is essentially the conditional copula function of C(u| V = v; Θ)CuV=vΘ.
Solution: As seen in the previous chapters, the bivariate Gumbel–Hougaard copula can be written as follows:

Then the hh function, i.e., h(u1, u2, θ)hu1u2θ, can be expressed as follows:

5.3.2 Vines
High-dimensional distributions have a significant number of possible pair-copula constructions. The regular vine, introduced by Bedford and Cooke (2001, 2002), is used to organize the general structure and embrace a large number of possible pair-copula decompositions. Two special types of regular vines, the C-vine and the D-vine (Kurowicka and Cooke, 2004), are given in the form of a nested set of trees and are used to decompose the multivariate density function. Figure 5.8 shows one sample specification corresponding to a five-dimensional D-vine that can be explained with Table 5.3.
Figure 5.8 A D-vine with five variables, four trees, and 10 edges.
Table 5.3. Five-dimensional D-vine.
Tree TjTj | Nodes | Edges |
---|---|---|
T1T1 | 1, 2, 3, 4, 5 | 12, 23, 34, 45 |
T2T2 | 12, 23, 34, 45 | 13|2, 24|3, 35|4 |
T3T3 | 13|2, 24|3, 35|4 | 14|23, 25|34 |
T4T4 | 14|23, 25|34 | 15|234 |