Abstract
Atmospheric dispersion is a very important process in air pollution. The use of tall stacks to minimize the impacts of air pollutant emissions reflected the saying that “the solution to pollution is dilution.” Although this saying turned out to be wrong for several reasons, including the cumulative effect of a large number of individual sources and the formation of secondary pollutants at large regional scales, atmospheric dispersion is nevertheless one of the key processes that govern air pollution levels. This chapter presents the fundamental processes of atmospheric dispersion, their theoretical basis, as well as the advantages and shortcomings of different types of atmospheric dispersion models.
Atmospheric dispersion is a very important process in air pollution. The use of tall stacks to minimize the impacts of air pollutant emissions reflected the saying that “the solution to pollution is dilution.” Although this saying turned out to be wrong for several reasons, including the cumulative effect of a large number of individual sources and the formation of secondary pollutants at large regional scales, atmospheric dispersion is nevertheless one of the key processes that govern air pollution levels. This chapter presents the fundamental processes of atmospheric dispersion, their theoretical basis, as well as the advantages and shortcomings of different types of atmospheric dispersion models.
6.1 General Considerations on Atmospheric Dispersion
Pollutants are dispersed, and therefore diluted, in the atmosphere as a result of turbulence. This turbulence covers a wide range of spatial scales, including large-scale eddies, smaller eddies, and so on until the dispersion of kinetic energy at the molecular scale. Lewis Fry Richardson (1881–1953), an English physicist, summarized this cascade of processes in a more poetic fashion:
“Big whirls have little whirls that feed on their velocity,
and little whirls have lesser whirls and so on to viscosity.”
Since it is not possible to represent turbulence in a mathematical deterministic manner, it is necessary to introduce approximate representations of the main processes involved. Because of the presence of the Earth’s surface, which creates an anisotropy in the lower layers of the atmosphere, it is appropriate to differentiate between turbulence in the vertical and horizontal directions.
Vertical turbulence results from processes mentioned in Chapter 4, which include mechanical turbulence and thermal turbulence. The latter depends strongly on the thermal structure of the planetary boundary layer (PBL); it is very large when the atmosphere is unstable and weak when the atmosphere is stable. Convection phenomena, which occur in the PBL as well as in the free troposphere, mix also air parcels (and, therefore, air pollutants) in the vertical direction, but they typically correspond to coherent motions and, therefore, are not treated in detail here.
Horizontal turbulence may be created by mechanical processes in the PBL, as well as by thermal processes. It is more challenging to parameterize the relevant physical processes, compared to those governing vertical motions. Therefore, its mathematical modeling can be difficult, particularly at very large spatial scales.
Turbulent processes can be addressed either through a lagrangian or an eulerian approach. In the lagrangian approach, one uses a reference system that moves with the mean wind and turbulent motions are studied with respect to that moving reference system. Therefore, one refers to relative turbulent diffusion, because it is relative to this reference system. Thus, the meandering of a plume, which may result from large-scale motions affecting the reference system, is not included. Relative diffusion corresponds to the instantaneous plume size, because averaging the plume size over time will correspond to including some of the plume meandering in the plume size. In the eulerian approach, the ensemble of turbulent motions is considered with respect to a fixed reference system. Therefore, one refers to absolute turbulent diffusion. Both approaches become equivalent when the lagrangian approach covers the full range of the turbulent eddies.
6.2 Lagrangian Representation of Turbulence
In the lagrangian representation of turbulence, one follows the statistical evolution of the distance between two particles (or molecules) emitted from the same source (Csanady, 1973). If a particle is present at the location x’ at time t = 0, its random motion may be represented with the probability density p(x − x’, t) such that p(x − x’, t) dx is the probability that the motion of the particle during time t leads to its arrival in a space dx centered at the location x. Then, the concentration of particles present at time t at the location x (i.e., the mass of particles within a volume dx) is as follows:
where S0 is the mass of particles emitted at time 0 at the location x’. The assumption of a random turbulent diffusion process leads to a gaussian distribution of the particle concentrations with a standard deviation σ and its maximum at the source location x’. In three dimensions, the standard deviation of the distribution of particles undergoing turbulent diffusion may be defined in one direction (here x) for the entire particle population as follows (assuming that the source is at x’ = y’ = z’ = 0):

Therefore, the standard deviation of the particle distribution in one direction is equivalent to the root mean square of the motions of the particles in that direction. If turbulence is uniform and isotropic, then: σx = σy = σz = σ. The distance traveled by a particle at the speed u during time t is:
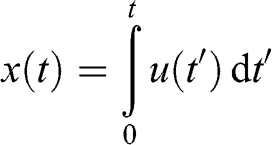
Therefore, the change in the square of the distance traveled as a function of time is as follows:
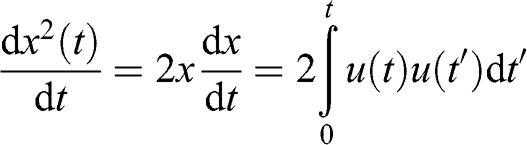
Taking the ensemble average for both sides of the equation:

The autocorrelation between the velocities of two particles at times t and t’ = t + τ□ is as follows:

Therefore, the change of the standard deviation as a function of time is written as follows (dt’ becomes dτL):

This expression is known as Taylor’s theorem (1922) (Geoffrey Ingram Taylor was a British physicist and mathematician). After integration:

Integrating by parts provides the solution for the square of the standard deviation of the distribution of particles for a steady-state dispersion process:

Thus:

where σ is the standard deviation of the particle distribution in one direction (σx in the mean wind direction, σy in the horizontal crosswind direction, σz in the vertical direction), t is the time since the emission, u is the wind velocity, and R(τL) is the lagrangian correlation function for a uniform and stationary turbulence. For such an idealized situation (constant in time and space), one may use a Markov process to represent the successive values of the velocity of the dispersed particles. (In a Markov process, the prediction of the future is performed using only information on the present state with no information on earlier states; although it is not entirely correct for a turbulent flow, it is a good approximation except for very short times.) Then, the correlation function may be represented by an exponential function:

where tL is the lagrangian time scale. It is defined as the integral of the correlation function:

The lagrangian time scale is on the order of 60 to 100 s in the planetary boundary layer. Near the source, i.e., as t —› 0, R(τL) —› 1 and the solution for the standard deviation is:

Therefore:

The standard deviation σ is proportional to the standard deviation of the instantaneous wind velocities, here σu, and to the time elapsed since the emission (or the distance traveled by the particle from the source if the mean wind velocity is constant, x = u t).
Far from the source, i.e., as t —› ∞, R(τL) —› 0 and the solution for the standard deviation is:

Let us define:

Then, σ2(t) may be written as a function of t, t0, and t1:

For an exponential correlation function, one obtains:
The value of t1 is obtained by partial integration:

Therefore:

And since t ≫ tL:

The standard deviation σ is proportional to the square root of the time elapsed since the emission or to the square root of the distance x traveled by the particle from the source if the mean wind velocity is constant (x = u t). Therefore, the lagrangian representation leads to a dispersion process that increases faster near the source (proportional to t or x) than farther downstream (proportional to t1/2 or x1/2). In his 1922 article, Taylor mentioned that this result was consistent with observations obtained in the laboratory and field observations of stack plumes. Indeed, the plume near the source displayed a conic shape (i.e., σ proportional to x), whereas farther downstream the shape of the plume resembled that of an elliptic paraboloid (i.e., σ proportional to x1/2). The change in the formulation of the standard deviation as a function of distance from the source is discussed further in Section 6.3.2, when the lagrangian and eulerian representations of turbulence are compared.
This lagrangian representation used the implicit assumption that the distribution of the instantaneous velocities about the mean velocity is gaussian. This assumption is generally appropriate for the horizontal directions; however, it is rarely verified in the vertical direction because the Earth’s surface leads to an important dissymmetry between upward and downward motions. Upward motions generally occur along narrow columns, whereas downward motions tend to occur with lower velocities and over wider columns of air.
6.3 Eulerian Representation of Turbulence
6.3.1 The Atmospheric Diffusion Equation
The continuity equation for an air pollutant (particle or molecule) is written similarly to that written for temperature in Chapter 4 (here, one does not account for emission, deposition, and transformation):

This equation is sometimes referred to as the atmospheric diffusion equation. Molecular diffusion is not taken into account here, because it is negligible in the atmosphere compared to turbulent diffusion, except near surfaces (see Chapter 11). Accounting for the turbulent nature of the flow, which affects not only momentum and temperature (see Chapter 4), but also the pollutant concentration:

Therefore, since u¯C‘ ¯=0 ; u‘C¯¯=0; etc. :

Using the continuity equation for momentum (see Equation 4.19) leads to the simplified equation:

Hereafter, as in Chapter 4, the following notations are used: C¯=C; u¯=u; etc. A first-order closure is performed by analogy with Fick’s law for molecular diffusion (Bird et al., 2006). For example, for the term u‘C‘¯
:

where Kxx, Kxy, and Kxz are the turbulent diffusion coefficients. It is generally assumed that the non-diagonal terms of the matrix of the turbulent diffusion coefficients are zero (Kxy = Kxz = 0). Then:

Similar terms are obtained for v‘C‘¯ and w‘C‘¯
. Note that the analogy with molecular diffusion does not have a true physical sense. Molecular diffusion is governed by the concentration gradient (the values of the molecular diffusion coefficient vary little among chemical species). In the case of turbulent diffusion, the term is also proportional to the concentration gradient, but it is the turbulent nature of the flow, which governs the importance of this term in the atmospheric diffusion equation. The term “dispersion” is typically used as synonymous of turbulent diffusion. The atmospheric diffusion equation is then written as follows:

Rewriting by grouping the advection and turbulent diffusion terms:

For the sake of simplicity, one assumes hereafter that the wind velocity and direction are constant along the x direction (i.e., v = w = 0), thus:

6.3.2 Instantaneous Point Source
The case study of an instantaneous point source, which was used for the lagrangian representation, is also used here first for the eulerian representation. Therefore, the initial and boundary conditions are as follows:

where S0 is the mass (g) emitted from the source, which is located at (xs, ys, zs), and δ is the Dirac function. For example, for δ(x – xs):

The solution of the continuity equation is the concentration in a puff emitted by the source:

This equation shows a gaussian distribution of the concentration. Introducing the standard deviations of gaussian distributions, σx, σy, and σz:

A simple comparison between Equations 6.33 and 6.34 shows that the eulerian turbulent diffusion coefficients are related to the standard deviations of the concentration distributions by the following relationships:
