Abstract
Meta-elliptical copulas are derived from elliptical distributions. Kotz and Nadarajah (2001) and Nadarajah (2006) made solutions of meta-elliptical copulas available. In this chapter, we will review the definition and probability distributions as well as other properties of meta-elliptical copulas.
7.1 Meta-Elliptical Copulas
7.1.1 d-Dimensional Symmetric Elliptical Type Distribution
In previous chapters, we have discussed symmetric and asymmetric (i.e., nested) Archimedean copulas for multivariate modeling (i.e., d ≥ 3d≥3). We have shown that (i) symmetric multivariate Archimedean copulas require that all the correlated variables share the same dependence structure, and (ii) the nested asymmetric multivariate copulas still require that some variables share the same dependence structure. Compared to the symmetric and nested asymmetric Archimedean copulas, the meta-elliptical copulas are more flexible than the symmetric (or nested) Archimedean copulas for modeling multivariate hydrological variables.
Following Genest et al. (2007), a d-dimensional random vector z (z = [z1, …, zd]Tz=z1…zdT) is said to have an elliptical joint distribution, i.e., ℰd(μ, Σ, g)ℰdμΣg with mean vector μ(d × 1)μd×1, covariance matrix Σ(d × d)Σd×d, and generator g : [0, ∞)→[0, ∞)g:0∞→0∞, if there exists a stochastic representation, as follows:
where r ≥ 0r≥0 is a random variable with the probability density function as
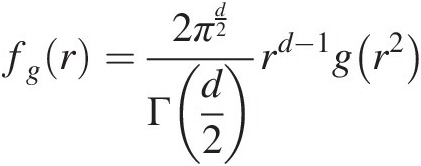
u (independent of r) is uniformly distributed on the sphere as follows:

A is Cholesky decomposition of Σ, i.e., AAT = ΣAAT=Σ
and the joint probability density function of z can be written as follows:

In Equations (7.1a) and (7.2), g(⋅)g⋅ is a scale function uniquely determined by the distribution of r and referred to as the probability density function generator. Common d-dimensional symmetric elliptical type distribution generators are given in Table 7.1.
Table 7.1. Common probability density function generators [g(t)gt] for elliptical copulas.
Copula | g(t)gt |
---|---|
Normal | 2π−d2exp−t2![]() |
Student | πv−d2Γd+v2Γd21+tv−d+v2![]() |
Cauchy | π−d2Γd+12Γ121+t−d+12![]() |
Kotza | sΓd2r2N+d−22stN−1exp−rtsπd2Γ2N+d−22s;r,s>0,2N+d>2![]() |
Pearson type II | Γd2+m+1πd2Γm+11−tm;t∈−11,m>−1![]() |
Pearson type VIIb | ΓNΓN−d2πmd21+tm−N;N>d2,m>0![]() |
Notes:
a Kotz type copula reduces to normal copula if N = s = 1, r = 1/2N=s=1,r=1/2.
b Pearson type VII copula reduces to Cauchy copula if m = 1, N = 3/2m=1,N=3/2 and reduces to Student copula if N=m2+1.
To build the meta-elliptical copula using the g(⋅)g⋅ function (listed in Table 7.1) and Equation (7.2), we should note that there is one limitation of these elliptical distributions, that is, the scaled variables z1σ11,z2σ22,…,zdσdd are identically distributed with the density function as follows:

and the CDF of the scaled variables given as follows:

From Equations (7.3) and (7.4), it is known that qg(x) = qg(−x)qgx=qg−x and Qg(x) = 1 − Qg(x) forx>0Qgx=1−Qgxforx>0.
Example 7.1 Derive the d-dimensional multivariate normal density function: z = [z1, …, zd]z=z1…zd.
Solution: As listed in Table 7.1, the probability density function generator for the multivariate normal distribution is gt=2π−d2exp−t2. Applying Equation (7.2), we have the following:

If μ = 0μ=0 in Equation (7.5), we have

By applying Equation (7.1), we have zTΣ−1z = r2(Au)TΣ−1(Au) = r2zTΣ−1z=r2AuTΣ−1Au=r2 and Equation (7.6a) may be rewritten as follows:

where
Σ=ρ11⋯ρ1d⋮⋱⋮ρd1⋯ρdd,ρii=1;∣ρij∣<1,i≠j;i,j=1,..,d, correlation matrix.
Example 7.2 Derive the d-dimensional multivariate Cauchy density function for z = [z1, …, zd]z=z1…zd.
Solution: Using the probability density function generator for the multivariate Cauchy distribution listed in Table 7.1:

Applying Equation (7.2), we have the following:

Similarly, if μ = 0μ=0, we have from Equation (7.7) the following:

Or equivalently

Without loss of generality, we will only investigate the case ℰd(0, Σ, g)ℰd0Σg. Let z = [z1, z2, …, zd]Tz=z1z2…zdT be a random vector with each component zizi with given continuous PDF fi(zi)fizi and CDF Fi(zi)Fizi. Suppose

where Qg−1 is the inverse of QgQg.
Then, the probability density function of z is given by
where the Jacobian matrix J is given as follows:

Since xi=Qg−1Fizi, we have ∂xi∂zj=dxidzi,i=j0,i≠j
. Rewriting matrix J, we have the following:

From xi=Qg−1Fizi, we have Fi(zi) = Qg(xi)Fizi=Qgxi. Differentiation on both sides leads to fi(zi)dzi = qg(xi)dxifizidzi=qgxidxi; dxidzi=fiziqgxi=fiziqg(Qg−1Fizi⇒J=∏i=1dfiziqgQg−1Fizi
Then, we have the following:

For x = (x1, …, xd)T~ℰd(0, Σ, g)x=x1…xdT~ℰd0Σg, we have the following:

Inserting Equation (7.11) in Equation (7.10), we have the following:

Using ℋℋ to represent the d-variant probability density function as

Equation (7.12) may be written as follows:

To this end, the d-dimensional random vector z is said to have a meta-elliptical distribution, if its probability density function is given by Equation (7.12). Denote x~ℳℰd(0, Σ, g; F1, …, Fd)x~ℳℰd0ΣgF1…Fd. The function ℋQg−1F1z1…Qg−1Fdzd is referred to as the probability density function weighting function. The class of meta-elliptical distributions includes various distributions, such as elliptically contoured distributions, the meta-Gaussian distributions, and various asymmetric distributions. The marginal distributions Fi.
can be arbitrarily chosen (Fang et al., 2002). The meta-elliptical distributions allow for the possibility of capturing tail dependence (Joe, 1997), which will be discussed later.
7.1.2 Bivariate Symmetric Elliptical Type Distribution
Suppose x~ℳℰ2(0, Σ, g)x~ℳℰ20Σg, we have the following:


Vector x has the following probability density function:

The marginal PDF and CDF of x are


A two-dimensional random vector (x1, x2)x1x2 follows an elliptically contoured distribution, if its joint PDF takes on the form of Equation (7.13). Its copula function can be written as follows:

where u=F1x1,v=F2x2,s=Qg−1u,t=Qg−1v.
The copula density function can be given as follows:
where

Now, let z = (z1, z2)T~ℳℰ2(0, Σ, g; F1, F2)z=z1z2T~ℳℰ20ΣgF1F2. Its probability density function may then be expressed as follows:

Take simple examples to illustrate the preceding.
Symmetric Kotz Type Distribution
Let x be distributed according to a bivariate symmetric Kotz type distribution. Inserting the density generator for Kotz type distribution (listed in Table 7.1) in Equation (7.2), we obtain the joint probability density function as follows:

where r>0, s>0, N>0r>0,s>0,N>0 are the parameters.
The marginal PDF (i.e., q1(x)q1x) can be written as follows:

The corresponding CDF (i.e., Q1(x)Q1x) can be written as follows:

Then, the copula probability density function can be given as follows:

Example 7.3 Show that the bivariate Kotz type distribution converges to the bivariate Gaussian distribution as noted in Table 7.1, i.e., N = s = 1, r = 1/2N=s=1,r=1/2.
Solution: Substituting N=s=1,r=12 into the probability density function generator of symmetric Kotz type distribution, we have

Comparing with the probability density function generator for the normal copula in the bivariate case, we have the following:

Now we show that the bivariate Kotz type distribution reduces to the bivariate normal distribution if N=s=1,andr=12. The same conclusion is reached for higher dimensional cases.
Example 7.4 Compute the copula density function for symmetric Kotz type distribution with the information given as N = 2.0, s = 1.0, r = 0.5, ρ = 0.1, u = 0.4, v = 0.3N=2.0,s=1.0,r=0.5,ρ=0.1,u=0.4,v=0.3.
Solution: Using Equation (7.22), we can calculate Q−1(u), Q−1(v)Q−1u,Q−1v numerically as follows:
Using Equation (7.20), we can compute the joint density function as follows:
Using Equation (7.21), we can compute the univariate density as follows:
Finally, substituting the computed quantities above into Equation (7.23), we have the following:

To further illustrate the shape of the bivariate symmetric Kotz type density function, Figure 7.1 graphs the bivariate density function for the following:
1. N = 2.0, s = 1.0, r = 0.5, ρ = 0.1N=2.0,s=1.0,r=0.5,ρ=0.1.
2. N = s = 1, r = 0.5, ρ = 0.5N=s=1,r=0.5,ρ=0.5: bivariate normal distribution.
Figure 7.1 Copula density plots for Kotz type bivariate distribution.
Symmetric Bivariate Pearson Type VII Distribution
The PDF of symmetric bivariate Pearson type VII distribution can be given as follows:

where N>1N>1, and m>0m>0 are parameters.
When N=m2+1, Equation (7.24) is the bivariate t-distribution with m degrees of freedom. When m=1,n=32
, Equation (7.24) is the bivariate Cauchy distribution.
The marginal PDF of symmetric bivariate Pearson type VII distribution is as follows:

The corresponding CDF of symmetric bivariate Pearson type VII distribution can be written as follows:

where x ∈ (−∞, ∞), m>0, N>1.x∈−∞∞,m>0,N>1.
Then, the copula density function c(u, v)cuv can be given as follows:

Example 7.5 Show the following bivariate Pearson type VII distribution cases are true.
Show the following cases are true:
1. N=m2+1
, the bivariate Pearson type VII distribution is the bivariate Student t-distribution with m degrees of freedom.
2. m=1,N=32
, the bivariate Pearson type VII distribution is the bivariate Cauchy distribution.
Solution:
1. N=m2+1
When N=m2+1
, the probability density function generator of the Pearson type VII distribution may be rewritten as follows:
g2PVII=Γm2+1Γm2−1πm1+tm−m2+1;N>1,m>0,N=m2+1
Comparing with the probability density function generator for the bivariate Student t-distribution g2t=πv−1Γv2+1Γv21+tv−v2+1
, we show that when N=m2+1
, the bivariate Pearson type VII distribution reduces to the bivariate Student t-distribution. The same conclusion is reached for higher-dimensional cases.
2. m=1,N=32
When m=1,N=32
, the probability density function generator of the Pearson type VII distribution may be rewritten as follows:
g2PVIIt=Γ32Γ12π1+t−32,m=1,N=32
Comparing with the probability density function generation for the Cauchy distribution g2Cauchy=π−1Γ32Γ121+t−32
, we show that when m=1,N=32
, the bivariate Pearson type VII distribution reduces to the bivariate Cauchy distribution. The same conclusion is reached for higher-dimensional cases.
Example 7.6 Compute the Pearson type VII copula density with the information given as follows: m = 0.5, N = 2.0, ρ = 0.1, u = 0.4, v = 0.3m=0.5,N=2.0,ρ=0.1,u=0.4,v=0.3.
Solution: Applying Equation (7.26), we can compute Q−1(u), Q−1(v)Q−1u,Q−1v numerically as follows:
Substituting
Q−1(u) = − 0.1443; Q−1(v) = − 0.3086Q−1u=−0.1443;Q−1v=−0.3086into Equation (7.24), we can compute the copula density function c(0.4, 0.3)c0.40.3 as c(0.4, 0.3) = 1.1941c0.40.3=1.1941.
To illustrate the shape of Pearson type VII distribution, we graph the Pearson type VII copula density function for the following parameters in Figure 7.2:
1. m = 0.5, N = 2.0, ρ = 0.1m=0.5,N=2.0,ρ=0.1.
2. m = 3, N = 2.5, ρ = 0.2m=3,N=2.5,ρ=0.2: bivariate Student t-distribution with degrees of freedom as 3.
3. m=1,N=32,ρ=0.2:
bivariate Cauchy distribution.
Figure 7.2 Pearson type VII copula density plots.
Symmetric Bivariate Pearson Type II Distribution
The PDF of symmetric bivariate Pearson type II distribution can be expressed as

where m> − 1m>−1.
The marginal PDF can be given as follows:

The corresponding CDF can be given as follows:

The copula probability density function can then be given as follows:

Example 7.7 Compute the bivariate Pearson type II copula density function with information given as follows: m = − 0.5, ρ = 0.1, u = 0.4, v = 0.3m=−0.5,ρ=0.1,u=0.4,v=0.3.
Solution: Applying Equation (7.30), we can compute Q−1(0.4), Q−1(0.3)Q−10.4,Q−10.3 numerically as follows:
Applying Equation (7.31), we can compute the bivariate Pearson type II copula density function as follows:
To further illustrate the shape of the bivariate Pearson type II copula density function, we graph the Pearson type II copula density function for the following parameters in Figure 7.3:
m = − 0.5, ρ = 0.1m=−0.5,ρ=0.1; (2) m = 0.5, ρ = 0.2m=0.5,ρ=0.2.
Figure 7.3 Pearson type II copula density function plots.
7.2 Two Most Commonly Applied Meta-Elliptical Copulas
In Section 7.1, we have stated that (1) the symmetric meta-Kotz type distribution reduces to the meta-Gaussian distribution if N = s = 1, r = 0.5N=s=1,r=0.5; (2) the symmetric meta-Pearson distribution reduces to the meta-Student t-distribution if N=m2+1. In this section, we will start to focus on the discussion of two most commonly applied meta-elliptical copulas, and these are meta-Gaussian and meta-Student t copulas.
7.2.1 Meta-Gaussian Copula
A d-dimensional meta-Gaussian copula can be expressed as follows:

where Φ−1(⋅)Φ−1⋅ represents the inverse function of standard normal distribution; ΦΣ(Φ−1(u1), …., Φ−1(ud))ΦΣΦ−1u1….Φ−1ud represents multivariate standard normal distribution function; ΣΣ represents the correlation matrix; Σ=1⋯ρ1d⋮⋱⋮ρd1⋯1,
ρij=1i=jρjii≠j,ρij=sinπτij2,τi,j the rank correlation coefficient;
d the dimension of continuous multivariate random variables; and w the integral matrix: w = [w1, …, wd]Tw=w1…wdT.
Let gw1…wd=12πd2Σ12exp−12wTΣ−1w,x1=Φ−1u1,…,xd=Φ−1ud, Equation (7.32) may be rewritten as follows:

and its copula density function can be given as follows:

or equivalently

Applying the partial derivative rule of inverse function,

In Equation (7.34), Φ(⋅)Φ⋅ is the CDF of standard normal distribution: Φx=∫−∞x12πe−t22dt; and ϕ(⋅)ϕ⋅ is the PDF of standard normal distribution: ϕx=12πe−x22
.
Now substituting Equation (7.34) back into Equation (7.32) or (7.32a), we can calculate the partial derivatives for the d-dimensional meta-Gaussian copula in what follows.
First-Order Partial Derivative
Using ∂C∂u1 as an example, the first-order partial derivative of the meta-Gaussian copula may be derived as follows:

Second-Order Partial Derivative
Using ∂2C∂u1∂u2 as an example, the second-order partial derivative of the meta-Gaussian copula may be derived as follows:

dth-Order Partial Derivative
Repeating the derivative d-times, we obtain the meta-Gaussian copula density function as follows:

Let ς = [x1, …, xd]T = [Φ−1(u1), …, Φ−1(ud)]Tς=x1…xdT=Φ−1u1…Φ−1udT. Equation (7.37) may be rewritten as follows:




Note that in Equation (7.38), ∏i=1de−Φ−1ui22 may be rewritten as follows:


Substituting Equations (7.38a) and (7.38b) into Equation (7.38), Equation (7.38) may be simplified as follows:

Recall that ςTς = ςTIςςTς=ςTIς, where I is d by d identity matrix. Equation (7.39) may also be rewritten as follows:

Example 7.8 (Bivariate meta-Gaussian copula): Compute the bivariate meta-Gaussian copula and its copula density function with the given information: Σ=10.20.21,u1=0.4,u2=0.3,
and show the first-order derivative of the bivariate meta-Gaussian copula.
Solution: Applying Equation (7.32) for d = 2d=2, we have the bivariate meta-Gaussian copula as follows:

From standard normal distribution, we have the following:

Substituting Φ−1(0.4), Φ−1(0.3), Σ−1Φ−10.4,Φ−10.3,Σ−1 and ∣Σ∣∣Σ∣ into Equation (7.40), we have the following:
Applying Equation (7.39a) for d = 2d=2, we have the meta-Gaussian copula density function as follows:
